
A team of US-based researchers has created an innovative deep-learning model that analyses CT scans and clinical information to predict six-month outcomes for patients with severe traumatic brain injury (TBI). In addition to outperforming the predictions of neurosurgeons, the algorithm can also accurately steer TBI patients towards life-saving care.
Better clinical decisions
As part of the research, data scientists at the University of Pittsburgh School of Medicine worked with neurotrauma surgeons at the University of Pittsburgh Medical Center (UPMC) to create a novel artificial intelligence model that processes multiple head CT scans of severe TBI patients. The algorithm, described in Radiology, also analyses patients’ vital signs, blood tests and heart function, as well as estimates of coma severity.
In recognition of the fact that brain imaging techniques evolve over time, and that image quality can vary substantially from patient to patient, the team accounted for data irregularity by training the algorithm on a range of different imaging protocols.
The researchers, led by co-first authors Matthew Pease and Dooman Arefan, validated their model by testing it on two patient cohorts – one consisting of more than 500 severe TBI patients previously treated at UPMC and the other of 220 patients from 18 institutions across the country, through the TRACK-TBI consortium. They compared the model’s performance with that of the IMPACT model and the predictions of three neurosurgeons.
The developed model could accurately predict patients’ risk of death and unfavourable outcomes at six months following the traumatic incident. Importantly, the model maintained its ability when tested on an independent multi-institutional cohort from the TRACK-TBI consortium. The model was also shown to outperform the predictions made by three attending neurosurgeons.
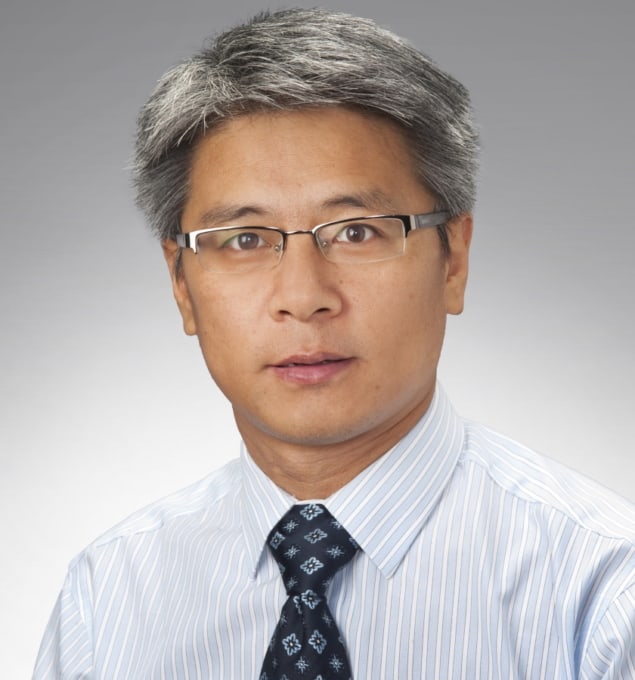
As senior co-authors Shandong Wu and David Okonkwo explain, TBI is a disease that disrupts normal brain function and can lead to permanent neurological, emotional and occupational disability. When treating such injuries, physicians rely on prognostication to guide clinical therapy, yet struggle to accurately prognose outcomes in severe TBI. As such, Wu notes, there is a “great need and potential to leverage multimodal clinical information and machine learning to develop data-driven prediction models to improve outcome prediction for severe TBI patients”.
“We used deep-learning and curriculum-learning techniques to develop prediction models that process both head CT imaging data and other clinical variables of patients,” says Wu. “In practice, this model can provide an automated prediction to an individual patient’s recovery potential to better inform clinical decisions and patient care.”
Individualized predictions
Wu observes that, in recent years, machine learning and deep learning have transformed medical data analysis and improved performance in supporting computer-aided detection diagnosis and triage of medical diseases. Indeed, many machine learning-based models and tools are now under academic investigation and clinical evaluation.
In Wu’s view, the key advantage of the new model is that it is capable of effectively analysing multidimensional and multimodal data, such as images and non-imaging clinical data, in an automated manner. This means that machine learning can learn essential information from these complex data, which may be difficult for a human physician to digest and process.
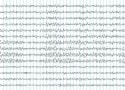
AI predicts coma outcome from EEG trace
“Our method can also provide individualized predictions compared with existing models such as the IMPACT model, which was designed to guide clinical trials and not prognose individual patients,” he says.
At present, the model is based on data acquired at a patient’s admission to the emergency room, but the project team plans to further enhance it by incorporating longitudinal data acquired during the course of the TBI patient’s care.
“We also plan to explore evaluation and identify potential barriers with regards to deploying such models in clinical workflow and settings,” adds Wu.
AI in Medical Physics Week is supported by Sun Nuclear, a manufacturer of patient safety solutions for radiation therapy and diagnostic imaging centres. Visit www.sunnuclear.com to find out more.